5 Key Trends Shaping the Future of Spend Analytics
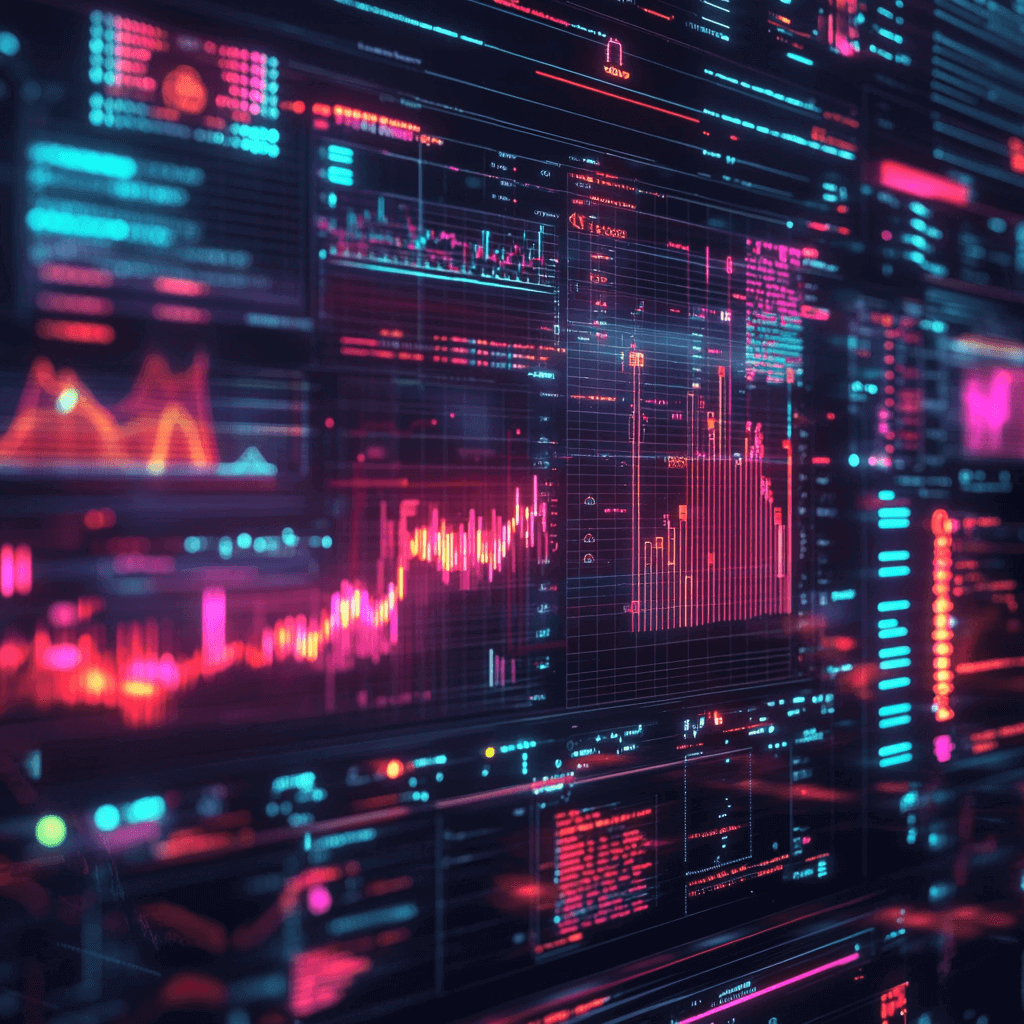
Spend analytics is a complex process of collecting, cleaning, analyzing, and reporting on procurement spend data.
If done right, it can be the key to greater spend visibility across the entire company and uncover hidden opportunities for cost savings.
And guess what?
Thanks to some exciting emerging technologies and trends, improving your spend analytics and unlocking those perks is now more possible than ever.
Today, we’ll be exploring these exciting innovations.
We’ll show you how to leverage them, reveal the many benefits they can bring, and even outline some real-life examples of companies already implementing these systems and practices.
Let’s get started and see what the future has in store for spend analytics.
AI has gradually entered all roles, processes, and tasks within finance and procurement, and spend analytics is no exception.
Did you know that, in a 2023 KPMG study, 60% of respondents believed generative AI would have the most significant impact out of all technologies in the upcoming 12-18 months?
Additionally, 71% agreed that this tech would be highly impactful in the next 3-5 years.
Illustration: Veridion / Data: KPMG
Is this surprising?
Not really.
AI, and its subset machine learning, are very powerful tools, particularly for spend optimization and analytics, and they are bound to get even stronger in the future.
But let’s talk specifics.
What does AI actually do in the context of spend analytics?
Here are a few applications:
Data Cleaning | AI improves data quality by removing bad data and detecting errors during manual entry based on other features of the transaction, such as vendor, material type, and supplier |
Data Categorization | AI automatically collects data from invoices, purchase orders, and other sources, classifying them into relevant spend categories |
Data Analysis | AI tracks historical data, performs internal and external benchmarking, and identifies factors impacting these numbers, such as changes in commodity prices |
So, AI doesn’t just collect and enrich spend data.
It can also analyze it and provide actionable insights, like spending patterns or cost-saving opportunities.
These insights can then be used, for instance, to renegotiate contracts with suppliers or consolidate vendors for better spend control.
Take Bass Pro Shops, for example.
After acquiring Cabela’s, another leading outdoor sports retailer, they found themselves with hundreds of millions in spend, but lacked the transparency needed to control it.
Where did all that money go and why?
Was there any room to cut some of these costs?
Nobody really knew for certain.
However, that changed after implementing an AI-powered spend analytics tool, says David Elford, their Director of Non-Merchandise Procurement.
Illustration: Veridion / Quote: Coupa
The solution provided better visibility into the benefits achieved through negotiations, gave them more control over their spend, and maximized their purchasing power in the process.
This is precisely why AI is so important in spend analytics.
It offers unparalleled transparency and fast, easy analysis—capabilities that no manual method could ever match.
Adnan Masood, Chief Architect of AI & Machine Learning at UST, a digital transformation solutions company, perfectly summarizes what AI, and machine learning in particular, are all about.
Illustration: Veridion / Quote: TechTarget
That’s right.
From accurate information to actionable insights to smarter decisions to better outcomes, AI makes it all possible, and it will undoubtedly continue to do so in the future.
The era of periodic analysis of historical spending data is fading away.
Now, decisions must be made practically on the go to keep pace with rapidly changing market conditions.
This is why we’re witnessing a shift towards real-time spend analytics.
Real-time analytics provide immediate insight into current cash flow, expenses, and revenue, so there’s no need to wait months—or even a full year—for financial reports.
Having access to a complete picture of your financial health at all times is extremely valuable.
It helps you spot opportunities, address risk, and keep you agile in general.
As Chad Gottesman, President and Board Member of the circular industry platform, Apkudo, notes, real-time data along with other tech can be an amazing value-driver for procurement.
Illustration: Veridion / Quote: Procurement Magazine
And he’s absolutely right.
With timely spend data, you can immediately identify issues like unauthorized spending, overspending, potential cash flow problems, and more.
Yes, these are issues that would’ve eventually been detected anyway, but likely only after they had spiraled out of control.
Real-time analytics, on the other hand, allows you to tackle them head-on before they become costly setbacks.
Microsoft is a good example of a company that employs this approach.
They broke down what their spend analytics solution can do:
“It can now review and risk rate its spend in every territory and business unit against all relevant controls in real time, pinpoint anomalies and high-risk transactions, and submit these for detailed compliance review and reporting.”
Holly Younggren, their Group Global Process Manager, explains this allowed them to shift from random to strategic, risk-based sampling and saved them quite a bit of time.
Illustration: Veridion / Quote: EY
They no longer have to blindly search for anomalies or non-compliance across thousands upon thousands of monthly transactions.
Instead, their system points out the issue as soon as it detects it.
They can then focus on fixing it and keeping their operations smooth and risk-free.
Overall, you can think of it like this: AI ensures data completeness and accuracy, while real-time analytics guarantees its timeliness.
Together, they provide the most reliable spend data you could ask for, empowering you to make smarter, faster decisions.
Predictive analytics takes all the spend data you’ve gathered and uses advanced statistical models and algorithms to analyze it and then make predictions about future outcomes.
This capability enables businesses to predict their future expenses, external market and pricing trends, and various other relevant (both qualitative and quantitative) insights.
As Amit Sharma, co-founder and CEO of data connectivity platform CData, puts it, such insights are very valuable.
Illustration: Veridion / Quote: Forbes
For example, Heineken uses predictive analysis to optimize their advertising spend.
The tech analyzes their rich external and internal spend data, taking into consideration the seasonality of certain beers as well as the duration, cost, and impact of previous promotions.
Based on this analysis, it comes up with a demand forecast, which the Heineken team can then use to determine when and how to adjust their advertising budget for specific promotions.
Given that they invest billions in this category, predictive analytics really comes in handy for them, helping them spend much smarter.
Now, if predictive analytics represents the third stage in the analytics maturity spectrum, prescriptive analytics is the fourth and final stage that goes hand in hand with it.
Source: Veridion
It does everything predictive analytics does but also goes a step further by providing advice on the best course of action based on the insights it derives.
Mick Hollison, Founder and CEO of the advisory and consulting firm RedLine Advisors, explains the difference between the two well.
Illustration: Veridion / Quote: ThroughPut
Solutions with prescriptive capabilities can identify value-creating opportunities and activities that mitigate risk all on their own.
For instance, they might alert you to move a portion of your critical spend away from that vendor with poor credit ratings.
This technology doesn’t rely on humans to spot correlations and patterns, so it rarely misses anything, which is particularly valuable in complex operations with large spend and many, many transactions.
All in all, these two technologies truly take spend analytics, as well as financial decision-making in general, to a whole new level.
And while many companies, especially smaller ones, are still in the early stages of analytics maturity, it’s only a matter of time before these advanced tools become the norm.
The insights they provide are simply too powerful to ignore.
Companies are starting to recognize that accurate spend analysis relies on both internal and external spend data and are, therefore, consolidating various data sources to get better results.
But what exactly do we mean by internal and external data sources?
Below, we break it down.
Source: Veridion
Relying on a single source type provides an incomplete picture of your spend, which can lead to inaccurate analysis and, in turn, missed opportunities and overspending.
For example, you might diligently monitor how much you pay your suppliers, but without monitoring the external market and pricing trends, you could miss the fact that a certain vendor is overcharging you.
If you had that information, you could’ve at least tried to renegotiate the contract to slash those costs.
Remember, opportunities for cost savings and strategy optimization are always somewhere out there, but to identify them, you need a full context of your spend.
So, to consolidate all their spend data sources, organizations try to build a single source of truth.
Spend management systems are commonly used for this purpose, but source-to-pay (or procure-to-pay) solutions, like the one you see below, are even more effective.
Source: PayHawk
These digital tools capture all possible spend data, compiling purchase orders, invoices, and requests in one place.
They can also flag discrepancies and match invoices or receipt notes with corresponding POs, making sure the information you work with is accurate and up-to-date.
With their customizable, multi-step approval workflows you can even prevent unauthorized spending and ensure all activities and reports are compliant with your spending policies.
Essentially, source-to-pay systems help track all company purchases and provide visibility across all commitments, including open requests and past or upcoming payments.
That way, you have access to most of the relevant data.
But there’s more.
Companies are also integrating these solutions with other tools they use, such as accounts payable software, payroll solutions, employee reimbursement systems, or CRM platforms.
Ideally, these integrations are achieved using the software’s native capabilities.
However, if native integration isn’t possible, tools like Zapier can help establish connections between various solutions.
Source: Zapier
The bottom line is this: the more interconnected your systems are, the better your spend data becomes.
That’s why there’s such a large emphasis on integration capabilities in procurement and finance software these days—they contribute to a much more reliable spend analysis.
Tail spend analytics are now enabling companies to maximize cost savings like never before.
Tail spend refers to all non-strategic, both high and low-volume transactions, as well as infrequent purchases made across various company departments, suppliers, and categories.
Using the 80/20 rule, tail spend could be described as that remaining 20% of spend that goes to 80% of suppliers.
Source: Veridion
It’s notoriously difficult to manage, but thanks to advanced spend analytics tools, that’s changing fast.
Organizations are finally discovering how improving tail spend management can provide them with better visibility into overall spending and unlock new cost-saving opportunities that might’ve otherwise gone unnoticed.
In fact, back in 2019, Boston Consulting Group found that digitizing tail spend management can reduce yearly expenses by 5 to 10%.
Illustration: Veridion / Data: BCG
That’s quite a significant amount, especially for larger enterprises with substantial annual expenditures.
Just take Boeing, for example.
Their tail spend was so huge that it was nearly impossible to monitor, let alone control.
And the fact that a lot of their RFQs and sourcing were done offline, via mail, and many other disconnected channels didn’t help.
Tiffany Andrews, Senior Manager at Boeing’s Indirect Procurement Center of Excellence, explains the issue in more detail:
“What we didn’t have was a good handle on our transactional procurements that we’re going through. We had catalogs in our systems, but anything that wasn’t on a catalog that was strategically placed was considered a SPO or a one-time and we didn’t have a great handle on that.”
However, their AI-powered spend management system easily fixed the problem, she says.
“What it would do is pull all of our trends from our old work system and our current system and [perform] an evaluation of all of our data, of our spending trends. How we like to spend our money and what suppliers that is with.”
It automatically aggregates and categorizes the data across all their locations, categories, and vendors, leading to greater transparency and more reliable spend analytics.
The solution also enables them to model and analyze various scenarios—like comparing how their spending would look with different suppliers— to slash their costs even further.
The lesson learned?
Don’t underestimate the power of tail spend analytics.
It can open the door to savings you never knew existed and completely transform your financial health.
We’re definitely bound to see more companies, both big and small, jumping on board and giving more attention to this often unmanaged, yet important category.
Overall, in the future, we’re likely to see even more use cases for AI in spend analytics.
Right now, it’s already doing an impressive job of cleaning, organizing, and analyzing data, but imagine the possibilities as it evolves to deliver even more powerful predictive and prescriptive capabilities.
Naturally, some people may be hesitant to embrace these technologies and let go of the tried-and-true methods they know so well.
That’s why, if you’re considering implementing anything you learned from this article, it’s vital to reassure your staff that these tools and practices are here to help them, not replace them.
And once you successfully introduce them, it won’t take long before your team experiences firsthand just how much easier and more efficient spend analysis and management can be.