The new paradigm for B2B data – Commercial Insurance Perspective
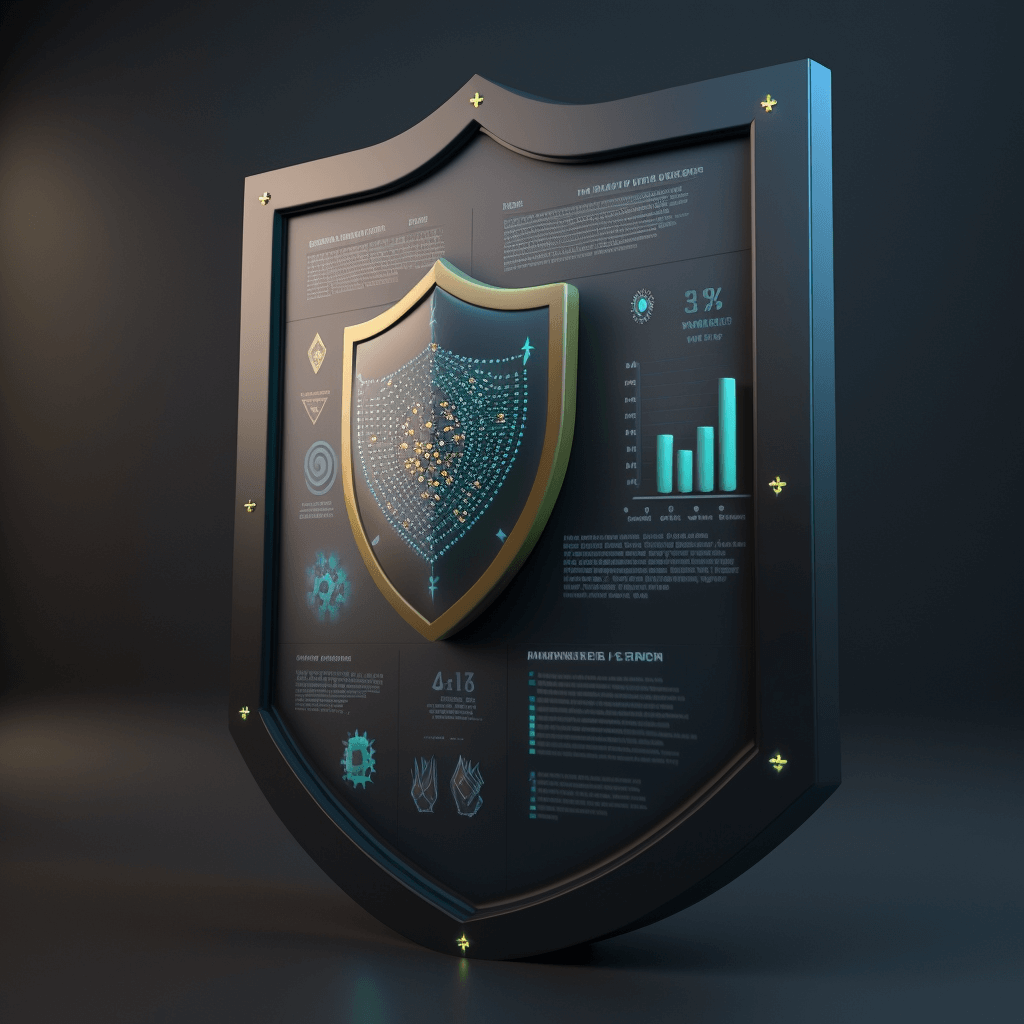
We love our data, and now that you're here, you're one step closer to loving it too.
A wide sample of data, so you can explore what is possible with our data
Choose ->
built with procurement in mind. Focused on manufacturers, products and more
Choose ->
built with insurance in mind. Focused on classifications, business activity tags and more
Choose ->
built with insurance in mind. Focused on classifications, business activity tags and more
Choose ->
built with procurement in mind. Focused on manufacturers, products and more
Choose ->
A wide sample of data, so you can explore what is possible with our data
A wide sample of data, so you can explore what is possible with our data
built with procurement in mind. Focused on manufacturers, products and more
built with insurance in mind. Focused on classifications, business activity tags and more
A wide sample of data, so you can explore what is possible with our data
built with procurement in mind. Focused on manufacturers, products and more
built with insurance in mind. Focused on classifications, business activity tags and more
built with insurance in mind. Focused on classifications, business activity tags and more
built with procurement in mind. Focused on manufacturers, products and more
Keep up to date with our technology, what our clients are doing and get interesting monthly market insights.
For the first time in history, we can directly trace massive problems to bad data and, at the same time, have the ability and the right tools to build systems that address these problems for the long run: high-quality data as infrastructure.- Florin Tufan, CEO @Soleadify
The way companies use data for making decisions is going through a massive paradigm shift that brings in a new wave of data solutions built by challenger start-ups that compete with multi-billion-dollar legacy players.
The aforementioned legacy players are currently stuck in the following scenario: Data used to be something humans would look at, analyze and make decisions. That required data to be simple enough for humans to read and interpret.
This is changing. Access to tools (e.g., Snowflake, Databricks etc.) and the advance of data science enable the transition of decisions through machine interpretation and automated processes, with data at their core. Data is now becoming part of the tech stack, deeply embedded in machine-powered decision engines across all business processes.
Billion-dollar data companies were built – and are still thriving – in the old paradigm. But they fail to deliver to modern requirements, so end users need to look elsewhere for better solutions. This is why alternative data (read “data from unofficial sources”) is growing at 50% CAGR YoY.
Remarkable examples of data being used at scale by leveraging big data solutions can be observed across multiple verticals.
🗺️Maps used to be something you looked at. Now, navigation apps crunch data and provide the fastest route. No human is looking at data.
🎯Ad targeting used to be based on people making assumptions of interests based on location, age, gender, and income level. Now, we have entirely automated ad-targeting solutions that analyze thousands of data points to match ads with eyeballs. No human is looking at age groups and assigning ads. Most advertisers have yet to learn which user traits make a user likely to engage with their ad.
If you work in insurance, you probably know that collecting data to plug into the actuarial models and price each policy takes time, money, and human capital.
In insurance, the shift towards using data as infrastructure for automation is already very clear:
Using data to automate insights, predictions, and decisions with minimal – if any – human supervision requires a new breed of data products delivered through powerful APIs that can be relied upon when plugged into processes and machine learning solutions, making or influencing trillions of dollars worth of decisions.
In this emerging world, detailed information about companies is highly relevant and needs to flow, as close to real-time, into all sorts of decision engines.
A restaurant’s cuisine or their policy on deliveries, whether a coffee shop sells CBD oils, the acquisition of a computer vision startup by an automotive corporation, a semiconductor factory’s product offering, a grain supplier’s warehouse locations, a plastic manufacturer’s certifications for its product lineup – these are all information that can heavily influence both individual decisions and sector/industry reports.
⏩Data updated in real time, instead of year-old data. Global coverage.
⏩Ever-growing depth, instead of shallow profiles.
⏩Extensive control over data transformation, instead of choices made for you
⏩Nuance, context and confidence scores around every data point instead of a simple value
Technical teams, and data science teams above all, need full control over choices and tradeoffs (e.g. from control over the tradeoff between accuracy and coverage, to requesting results using a specific taxonomy over another). Without the context crunching that comes from aggregating tens of millions of sources, it is nearly impossible for data vendors to really offer the control their clients demand.
💡 Consider the following two scenarios:
a. We’re evaluating a supplier located and incorporated in the US
b. We’re evaluating the same supplier, located and incorporated in the US, except that we now know they used to only manufacture in Russia until 12 months ago, and even more, they used to only operate in Russia 36 months ago
⏩Powerful APIs to enable easy consumption across many complex use cases
a. Provider A returns a list of products manufactured by 4M manufacturers in the world. In this case, the client now needs to embark on a 12mo+ journey to build a search engine on top.
b. Provider B exposes an API that gets as input a product name and returns a list of relevant manufacturers. The search engine is the API.
The results, in option (b), are much more accurate than the client would ever manage to get, because:
The current market leaders in B2B data act most of the time as a middleman between users and government provided data. The major flaw for this type of approach is that government records don’t represent the “truth” for what a business actually does, and their main purpose is regulatory compliance. It’s great for (parts of) AML and KYC but virtually useless for anything else.
We created the first alternative to the age-old source of truth in B2B data (government records), by turning the vast, unstructured content on the web into a dynamic data set on private and public companies.
Our main approach for tackling this challenge was to build a data factory that transforms real world business activity into a “source of truth” data set on organisations. This data (our product) is exposed through various APIs (our packaging) that cater to different stages of a wide array of B2B processes.
The stepping stone of this factory is a Google-like approach to sourcing data – making sense of the unstructured content on the web.
a. it infers missing data by making correlations between different signals and looking for similar companies across our data set
b. decides the truth between conflicting sources
The value of data is judged by coverage (how much of the universe it covers), depth (how much detail), veracity (how true and how recent) and usefulness (what problems can I solve with it). We look at what makes us different through these 4 lenses.
✅Coverage
We can truly say that we have a global covarage with a multilingual data sourcing infrastructure that supports 20 languages. We currently track 70M companies worldwide. With higher covarage for regions like North America, Europe, Australia and New Zealand. For now one of the main points to imrove on is expanding covarage accounting for distinct cultural differences that influence content processing. (A good example is China, that is currently only partially covered by our global models). Full coverage here
✅Depth
For now the actual depth of our data can be measured by the 50+ data attributes that we track for all the 70 million company profiles. “For now” is the term we use here, because they are constantly evolving and as we discover new sources, and new ways to add data points, and improve accuracy for existing ones this figure is subject to constant changes. One good example for this type of volatility is how we first time extracted our product data, through the span of 1 mouth we managed to extract 300M products from 4M manufacturers across the world. For a better understanding of our data points you can acces our Data Dictionary.
✅Veracity
In the space of data science, “veracity” refers to how accurate or truthful a data set may be. To achieve a level of high veracity for our data we enforce three fundamental principles.
✅Usefulness
We look at the usefulness of our solutions through three different perspectives:
Acces to in depth B2B web captured data can be the stepping stone for the next generation of commercial insurers. By leveraging this type of data, insurance companies can build seamless experiences for commercial lines and serve customers at scale with minimum risks.
Building the framework for this kind of end-to-end experiences is quite a difficult challenge. But in the long run it will make the difference between the players that lead the market and the ones that struggle to survive.
Let’s discover together how Soleadify’s data can help your company streamline commercial insurance underwriting.