5 Best Practices for Mastering ESG Data Management
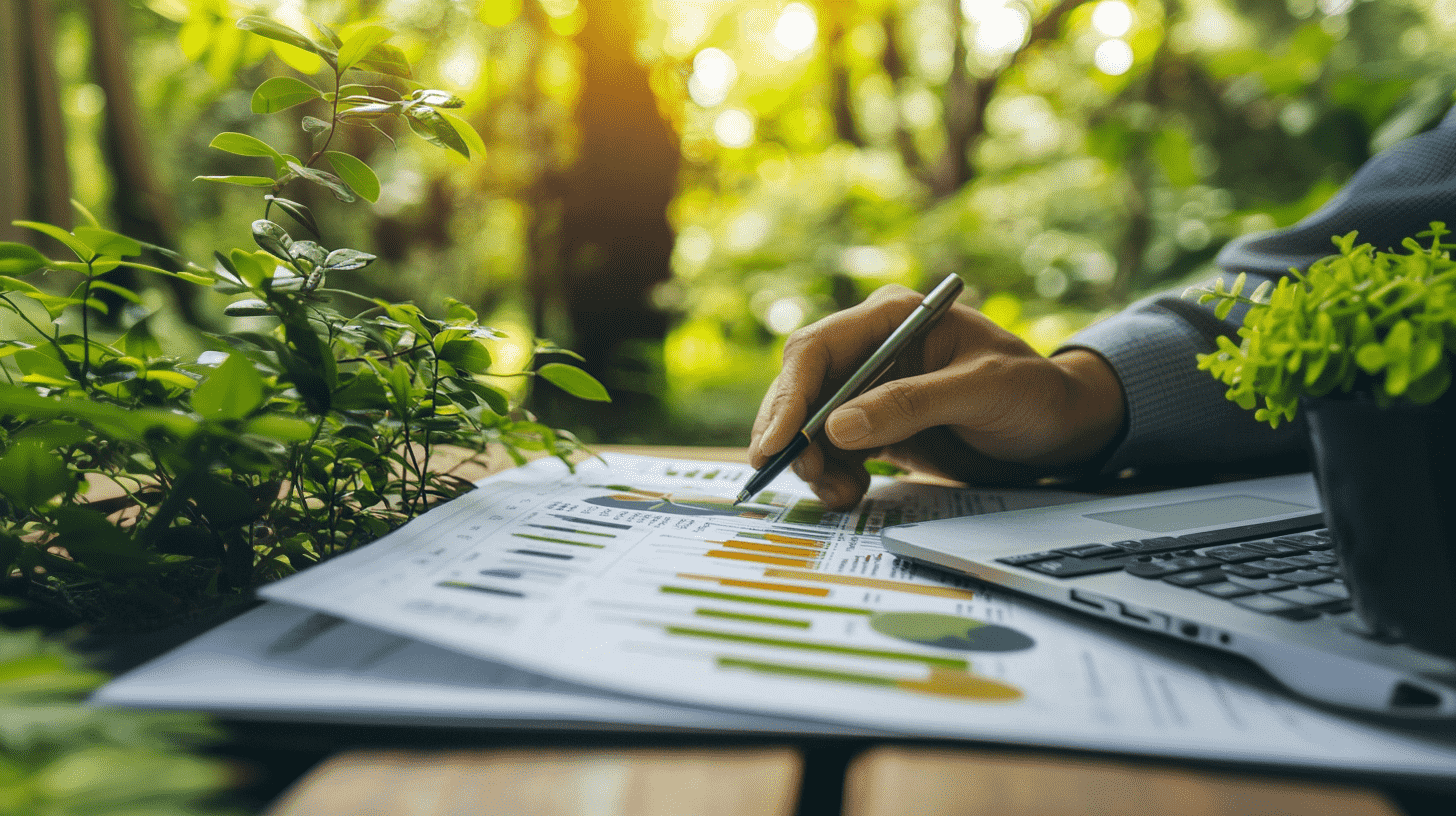
Key Takeaways:
Sustainability—consumers demand it, investors prioritize it, and regulations require it.
As a result, managing environmental, social, and governance (ESG) factors and reporting on them has become a top priority for businesses.
But without efficient ESG data management, achieving real impact and staying compliant is challenging.
In this article, we’ll explore five best practices to help you streamline ESG data, ensure compliance, and drive meaningful value for your organization.
Considering the scope and complexity of ESG factors that need to be managed, monitored, and reported, optimizing data collection processes is crucial for mastering ESG data.
This best practice stems from the vastness of ESG topics and the variety of sources from which data is collected.
As a reminder, ESG data encompasses:
While these examples only scratch the surface, they underscore the challenge of managing such diverse and expansive data.
As Iyngaran Panchacharam, a sustainability tech and AI expert, puts it:
Illustration: Veridion / Quote: LinkedIn
He continues to say that ESG data should be considered a data “ecosystem.”
However, this ecosystem consists of a wide range of disparate sources, often leading to fragmented, inconsistent, and hard-to-verify data.
That’s why establishing structured and scalable data collection frameworks is a critical first step.
This process involves defining how ESG-related data will be collected, stored, and validated to ensure it is trustworthy, actionable, and aligned with reporting requirements.
Here, automation, APIs, and verified third-party datasets play a key role in minimizing manual errors and ensuring data coherence and reliability.
For instance, our data engine, Veridion, leverages advanced AI technologies to gather accurate, comprehensive, and real-time ESG insights on suppliers globally.
Source: Veridion
ESG data is collected and updated weekly for all companies with an active online presence, currently covering over 123 million companies across 250 geographies.
This data can be exported in multiple formats or accessed via API integration, enabling seamless real-time updates within your existing business systems.
Since the data is sourced from publicly available information and verified for accuracy, it serves two key purposes:
This brings us to another critical aspect of optimizing ESG data collection—balancing primary and secondary data sources.
To illustrate, here’s an overview of what companies typically consider primary and secondary ESG data sources.
Source: Veridion
The first step in balancing ESG data collection is assessing its reliability.
For example, if a supplier claims to uphold fair labor practices, has this been verified through a site visit or cross-checked against regulatory and news reports?
Distinguishing between verified (directly collected or third-party validated) and unverified, self-reported ESG data is critical for both accurate reporting and effective strategic sourcing.
Another challenge is data standardization.
While fragmented primary data can hinder comparability, secondary data often lacks granularity, leading to potential miscalculations.
To address these issues, companies should establish clear, structured data collection processes that ensure accuracy, reliability, and compliance with reporting requirements.
Beyond collection, centralizing ESG data in one system is another essential step toward effective ESG management.
Efficient ESG data management starts with centralization.
Yet, many companies struggle with fragmented information spread across multiple systems, formats, and sources.
Without unified, integrated data access, ESG reporting is inefficient, error-prone, and non-compliant.
Moreover, siloed data makes it difficult to track ESG progress, verify supplier claims, and make informed decisions.
But this challenge isn’t unique to ESG data, a 2022 Informatica survey on data fragmentation found.
Illustration: Veridion / Data: Informatica
Such a high percentage of fragmented data management and the sheer number of data sources highlight the challenges of non-centralized ESG data environments.
Without a single source of truth, organizations face duplicate, inconsistent, or outdated information.
Naturally, this makes it difficult to ensure compliance, assess supplier ESG performance, and generate reliable reports.
To overcome this, businesses should implement centralized ESG data platforms that integrate multiple sources into a structured, easily accessible system.
While these platforms can pull data from ERP and procurement systems, a master data management (MDM) solution like HICX provides a dedicated, consolidated hub for supplier data.
Source: HICX
As shown, this supplier data consolidation tool allows you to import data from various ERP, P2P, and other systems into a single master database.
Remember, centralized supplier data serves a dual purpose—it supports both strategic sourcing and ESG reporting.
With all supplier information in one place, procurement teams can assess supplier capabilities, compare vendors, and identify sourcing risks more effectively.
They can also evaluate suppliers’ ESG factors based on their sustainability performance, ethical practices, and regulatory compliance.
Additionally, relevant data can be seamlessly extracted from the MDM system into an ESG data hub.
Here’s an example of one such platform designed for streamlined ESG reporting.
Source: Plan A
Combined with a centralized system, such tools enable quick extraction of relevant ESG data, ensuring accurate and timely ESG disclosures.
By integrating supplier data management, organizations eliminate data silos, enhance transparency, and streamline compliance with evolving regulations.
This centralized approach simplifies ESG reporting while strengthening the entire supplier sourcing process, from capability assessments and risk evaluations to sustainability tracking.
Overall, with all ESG data in one place, your company can drive data-backed ESG initiatives and ensure streamlined, reliable reporting.
To ensure ESG data accuracy, accountability, and compliance, companies need a strong governance framework.
This framework establishes:
Without these elements, organizations risk relying on inconsistent or unreliable ESG data, leading to poor sourcing decisions and ineffective supplier management on an operational level.
As for official sustainability reporting, data inconsistencies can result in reputational damage or regulatory penalties.
To mitigate these risks, many companies implement multi-step governance processes to uphold data integrity.
Below is an example of how PepsiCo structures its ESG data governance review to ensure consistency, accountability, and compliance.
Source: Pepsi
As illustrated, before any data is approved for ESG reporting, it undergoes five levels of verification and validation to ensure accuracy and compliance.
The steps are summarized in the table below:
Step | Action |
Sustainability Office Review | A dedicated Data Governance team reviews data files to ensure alignment with documented sustainability data governance methods. |
Subject Matter Expert Certification | Experts at various levels verify the completeness and accuracy of the data, ensuring accountability across all sustainability goals. |
Corporate Control Review | The Corporate Control team reviews sustainability data to confirm it aligns with financial and other corporate claims. |
Legal Review | Legal experts examine proposed disclosures to ensure both accuracy and compliance with regulatory requirements. |
Disclosure Committee Review | Senior leadership and the Disclosure Committee review final reports before publication to ensure consistency and compliance. |
This multi-step process helps ensure that ESG data is rigorously verified, reliable, and ready for transparent reporting.
However, the work doesn’t end once the data is validated.
Robust data governance requires continuous monitoring to adapt to evolving corporate sustainability goals, ESG regulations, and global frameworks.
Ongoing compliance with changing ESG standards can be maintained through regular reviews, audits, and updates to the data governance process.
Put simply, your governance framework must remain flexible and responsive to change.
Ultimately, such robust data governance framework is the foundation for mastering ESG data management.
Ensuring high-quality ESG data is crucial for maintaining credibility in sustainability reporting.
However, accurate data—both internal and external—is also essential for effective supplier selection, management, and risk mitigation.
Poor data quality can significantly undermine decision-making, compromising both ESG compliance and operational efficiency.
This can lead to inaccurate disclosures and missed opportunities for strategic sourcing.
With current uncertainties in global supply chains and evolving ESG regulations, the need for accurate, timely, and consistent data has never been more apparent.
The foundation of strong data quality and effective ESG reporting lies in ensuring coherence across key data quality dimensions, as illustrated here.
Source: Research Gate
Each dimension plays a crucial role in ensuring data quality, and together, they form a robust framework for reliable decision-making and accurate ESG reporting.
So, how do organizations ensure data quality across these dimensions?
By establishing clear data collection, validation, and review practices—while also leveraging automated data management solutions.
For example, a centralized MDM system, as mentioned earlier, can automatically flag missing, inconsistent, or outdated data—helping maintain data quality across key dimensions.
Additionally, trusted third-party sources can validate data accuracy and ensure ESG data remains up to date through regular updates.
A strong example is PepsiCo’s use of Veridion for external ESG data collection and validation within its data governance process.
Source: Veridion
Beyond leveraging automated data consolidation and validation tools, ensuring trustworthy ESG data also requires regular audits.
Audits help verify that all relevant data is captured, including ESG-specific inputs from suppliers and other stakeholders, while ensuring compliance with evolving standards.
Robust exception management procedures are also important.
These predefined, often software-enabled workflows help teams identify, review, and resolve discrepancies or anomalies in ESG data.
By automating alerts and corrective actions, they enable timely corrections, prevent data inconsistencies from spreading, and maintain overall data integrity.
When an organization implements all these tools and processes, the result is high-quality ESG data that drives reliable decision-making and ensures credible reporting.
Aligning internal ESG key performance indicators (KPIs) with globally recognized reporting standards is essential for ensuring credibility and comparability in sustainability reporting.
By mapping internal metrics to established frameworks such as GRI, SASB, CSRD, and CDP, organizations can stay ahead of evolving compliance requirements and benchmark their performance against industry standards.
This strengthens transparency and enables procurement teams to engage more effectively with suppliers, mitigate risks, and achieve ESG goals.
To better understand these frameworks, here’s an overview of the key elements of four major ESG reporting standards, courtesy of Traace:
Source: Traace
As shown, most sustainability reporting frameworks are voluntary, except for the EU Corporate Sustainability Reporting Directive (CSRD).
The standards (ESRS) under CSRD are mandatory for EU companies and non-EU firms with over €150 million in EU sales, and their implementation began in 2024.
Other frameworks may be required by investors or mandated by certain countries, such as the ISSB (International Sustainability Standards Board), which is being adopted by jurisdictions like the UK and Brazil.
So, to ensure compliance and comparability, companies should strategically map their internal ESG KPIs to relevant standards.
Since organizations often report across multiple frameworks, interoperability mappings help align ESG data with different reporting categories.
As CSR & ESG expert François Tréfois explains, these mappings ultimately enable ESG software to:
Illustration: Veridion / Quote: Traace
Essentially, software with built-in interoperability features allows the collected ESG data to be matched with relevant categories in each type of report.
This significantly simplifies both KPI alignment and reporting compliance.
To achieve this, companies should first identify the most relevant standards for their industry and stakeholders.
Conducting a gap analysis can then help assess whether existing ESG data meets reporting requirements or needs refinement.
Ultimately, aligning ESG metrics with recognized standards ensures compliance, enhances comparability, and improves reporting quality.
On an operational level, it also supports more informed procurement decisions that align with your company’s ESG goals.
Strong ESG reporting starts with efficient data management.
By optimizing ESG data collection, centralizing it for consistency, and enforcing robust governance, companies build a reliable foundation for sustainability reporting.
Prioritizing data quality ensures accuracy and credibility, while aligning ESG metrics with global standards enhances compliance and comparability.
Combined with the right tools and methods, these best practices ultimately support informed decision-making and strengthen procurement strategies that align with corporate ESG goals.